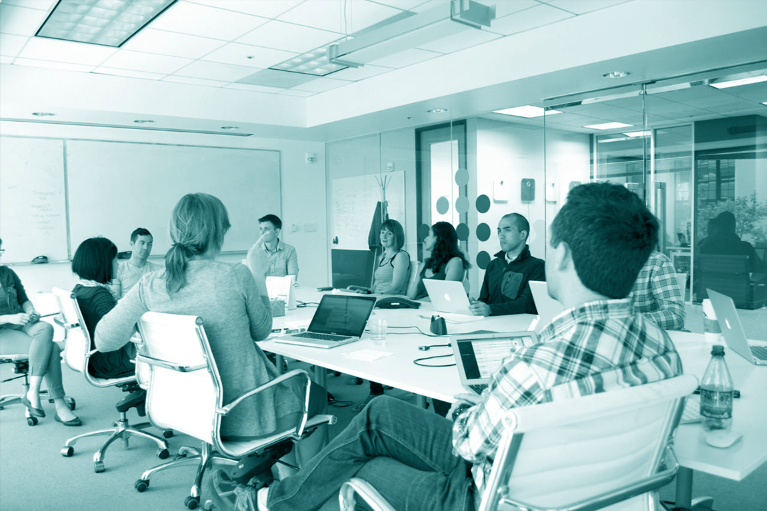
Fitbit的数据科学团队如何缩放机器学习
在拉吉·班恩(Raj Bhan)受伤的时候,他决定与Netflix的数据团队分道扬way。他一直在半程马拉松比赛中训练,并从网络上拔出了一项方案。不幸的是,他将自己归为错误的水桶,伤害了他的腿。
“That struck a chord,” he says. “People can suffer adverse effects from being in the wrong program at the wrong time.健身计划确实需要个性化。”
不过,拉杰受伤有一首诗。现在,他专门创建这些程序(有点)。拉杰(Raj)领导一个数据科学团队Fitbit, the consumer technology company most famous for its stylish wearables.
Ingesting users’ fitness and workout data, Fitbit devices report data back to users through its suite of apps. In the case of personal training tools like Fitstar, Fitbit’s apps can even make fine-tuned fitness recommendations. And that’s no small thing.
“Now that Fitbit trackers are ubiquitous in the market and we’re capturing data from millions of individuals, we are leveraging machine learning to provide smart guidance as part of a personalized experience,” Raj says.
In retrospect, the fitness data that a Fitbit logs probably could’ve helped Raj assess himself better than a standardized regimen. But even then he probably would’ve needed a more personalized service. Now, in a如果…-style twist, the algorithm that Raj’s team has built is the exact thing that could’ve ramped him for his race.
它是2017年。机器学习不是数据团队的虚荣项目。相反,它已成为最大的价值提供者之一。具有可用资源的团队需要确定其产188金宝搏备用网址品中的点,使机器学习可以强大的杠杆作用和专门创新。
菲比特的好问题
Fitbit’s product has a very good problem. The amount of data it tracks can be overwhelming. The devices ingest fitness data and feed it into the applications, where it’s paired with data from the user’s app interactions.From these data sets, the data science team can build a comprehensive user profile.不过,有职业危害。您获得的信息,您可能会失去清晰度。
“The volume of data does makes it challenging,” Raj says. “We have to make sure that we’re scaling both our hardware and our ETL processes.存储本质上是解决问题的问题,因此我们将精力集中在计算时间和处理上。”
Theoretically, sampling data sets would be a safe bet for a model. After all, Fitbit takes in a lot of data. But Fitbit’s product is user-centric. So long as it’s trying to build something precise to each user’s profile, there’s danger in recklessly sampling.
“We never know what we’re going to need later,” Raj says. “If we’re sampling 30%, there’s a potential for losing 70% of the things that are happening. In that case, our model can only be as good as what 30% of the data tells us.”
30%的样本在方向上是正确的;但是,一个方案也从网络上脱颖而出。
“我们一直在问我们还能使用用户数据做什么?” Raj says. “Offering more in the way of personalization and guidance is what we’re striving for.”
说起来容易做起来难。健身数据的财富为他们在用户面前的算法增加了一个具有挑战性的新维度。
但是,Raj的团队认为设备数据将提供明显更好的体验,试图在他们的机器学习实验中利用越来越多的它来改善这种体验。And they’re confident it’ll pay off.
The last mile
尽管机器学习应该是任何数据团队的核心竞争力,但建立模型不应是任何人都做的一切。那不是战略性的。最有效地利用机器学习的一部分是知道什么可以重新利用。需要创新的地方;以及可以提取最大价值的地方。
“The need to have people just cranking away at algorithms in house is being diminished,” Raj says. “Many third party companies out there provide these one-size-fits-all solutions that are good for probably 80% of solutions, right out of the box. If you really want to hone in and get that last 20%—到最后一英里- 那是您需要内部人员来解决这类问题的地方。”
Running that last mile can be significant. That’s why pays to do it in the right direction.
很少有人会不同意个性化是消费技术的未来。问题总是到什么程度.
The original Fitstar algorithm took post-workout feedback and rejiggered the intensity of the next workout accordingly. Users would input their feedback, Goldilocks-style:这太难了,太容易了,正确. The personal training app could, for example, scale the amount of push-ups up or down. Incrementally improving the product like this, the data team saw jumps in engagement.
But was it the best value the team could provide for all the fitness data a user was trading? Raj thought no.
He knew the next big algorithm his team invested in would have to make evermore significant strides in the arena of personalization. If his team was going to run that last mile, users had better feel it, too. So, they turned to the greatest and most difficult resource at their disposal—all that fitness data in user devices.
每个用户的模型
“What we’ve done with the latest iteration is truly integrated Fitstar data with Fitbit device data,” he says. “So, whether users have a proclivity toward cycling or running or using the elliptical or hiking, Fitbit automatically tracks those preferences and uses them to generate a custom workout for the user.”
Instead of relying solely on what users report to the app, the app makes its calls based on what the Fitbit device itself is saying. If you go cycling a lot, the algorithm will pick up on that signal and create leg-intensive workouts for you. But if you’d just gone cycling the day before, the algorithm knows you might need recovery time and will create an upper-body workout to give your legs some rest. It takes cognitive load off the user while building a more personalized workout.
拉吉(Raj)在一个不人物的疗法中的早期不幸事件使他教会了定向正确性的危险。原始的Fitstar算法为此解决了,但最新的算法代表了对个性化的真实投资。它不是要求您将自己整理成一个水桶,甚至不是建议精确的水桶。它创建了一个独特的适合您。
拉吉说:“归根结底,这是Fitbit的真正价值在于试图提供这种动机和健身改变体验的地方。”“我们希望提供见解,但是随着我们朝这个方向发展,我们希望提供指导。在这两件事之间,Fitbit在其他健身追踪器和类似的公司只是提供数据的地方取得成功。”
甚至像Fitbit这样的资源良好的数据团队也必须明智地选择:在各处进行创新,您的客户可能永远不会注意到。选择一个有意义的地方,每隔一段时间构建,客户会感觉到您的产品的差异。
Despite its massive scale, Fitbit is creating a product experience that maps to each individual user, and machine learning is the most effective way of utilizing the data at its disposal.
拉吉说:“每个人都不同,每个人都有不同的目标。”“这就是机器学习变得非常重要的地方,我们尝试为每个特定用户建立一个模型。”
从个人经验来看,他知道这有多艰辛和有多重要。因此,他将团队的努力集中在正确的项目上。尽管该产品比Raj加入时更聪明,更强大,但他的目标仍然相同,可以在正确的时间让每个Fitbit用户进入正确的程序。